In the public transport sector, data accuracy is a key factor in efficient management. At CITiO, we want to optimise this accuracy through denoising, an advanced method that is integrated into our Transport Analytics and Rail Analytics tools and enables our Fraud Tracker to be used.
What is denoising?
Denoising is an algorithmic method designed to correct inconsistencies and errors in passenger counting data that are also called ‘noise’. This method takes into account various anomalies, such as discrepancies in the numbers of entries and exits or errors due to counting sensor failures. It works on the principle of algorithms that analyse past and current data, including ticketing information, to produce a more accurate estimate of passenger numbers. This method is based on studies by Spanish researchers published in the journal of Applied Mathematical Modelling. Our team of Research and Development (R&D) experts have further developed this method by taking into account past counting data to identify trends and compare them with ticketing data.
The denoising process explained
The denoising process begins with the identification of inconsistencies in the data collected. For example, denoising should be carried out if:
- the total number of boardings is not the same as the total number of alightings on a given route;
- the number of alightings exceeds the on-board occupancy;
- the boardings, alightings or the occupancy exceed the vehicle’s maximum capacity at a given time.
Our algorithm then adjusts this data based on optimisation models aimed at removing existing inconsistencies. This involves slightly changing the counting sensor data, taking into account the usual occupancy trends and applying specific constraints to ensure the balance and plausibility of results.
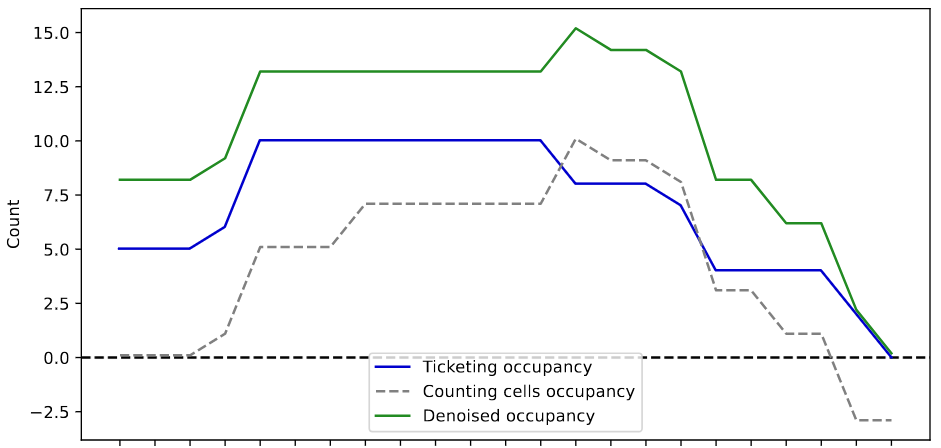
The Orléans case study: successful application of denoising
In the city of Orleans, the use of Transport Analytics and the accompanying denoising calculation method resulted in an accurate, real-time analysis of public transport ridership; a valuable aid for optimising transport services, right down to individual journey level. This tool provided transport managers with detailed information on passenger flow patterns, a valuable aid for optimising transport services. For more information on this partnership, see our case study in Orléans.
The importance of denoising
Biased counting data can lead to significant errors in public transport planning and management. For example, if counting regularly underestimates the number of passengers on a popular line, this can lead to insufficient allocation of resources, causing vehicles to be overcrowded and negatively affecting user experience. Conversely, overestimation can lead to over-allocating resources, unnecessarily increasing operational costs. This is why denoising is an effective way of mitigating this kind of situation and correcting counting data for more operational accuracy.
Denoising is integrated into our Transport Analytics and Rail Analytics solutions to provide the most reliable ridership data. By removing these inconsistencies, these tools ensure better understanding of public transport dynamics, which is essential for effective management. In our Fraud Tracker solution, denoised attendance indicators are used in conjunction with ticketing attendance indicators to accurately estimate fare evasion, enabling prediction of on-board fare evasion and analysis of fare evasion on the network.
Conclusion
Denoising is an important development for data accuracy in the public transport sector. At CITiO, we are proud to be implementing this new technology that will give operators and decision-makers the information they need to manage passenger flow.
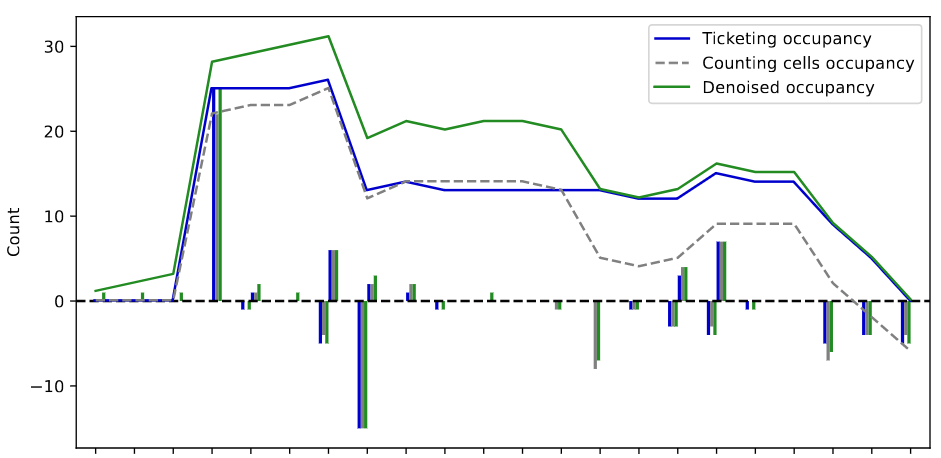
Ascending vertical bars represent boarding, descending vertical bars represent alighting. In blue, boarding/alighting and ticketing occupancy, in dotted grey, boarding/alighting and gross occupancy count and in green, boarding/alighting and denoised occupancy.